

- #Lisence key chronos soho notes how to#
- #Lisence key chronos soho notes update#
- #Lisence key chronos soho notes software#
- #Lisence key chronos soho notes crack#
This new machine learning stack required all sorts of new application code. Predicting where poop might be turned out to be a problem that was not just tricky from the analytical dimension. The goal was to give users an even more powerful view of the universe of possible locations routes unobstructed by poop. In the evolved version of the Dookie platform, built with the help of the new data science team, the app actually attempts to predict potential hotspots before they’re reported. Purple poop represents a location predicted to have dog poop The folks at Dookie are dog lovers who want to build a global social network around spotting and reporting rogue dog poop.
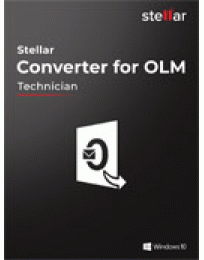
So, we’re going to imagine a hip startup in SoHo with a shoe-saving app named Dookie. For us New Yorkers, the prospect of walking out each morning and stepping right into a steaming pile is very real. In my analysis, I’m going to use a hypothetical application designed to solve one of the most pressing problems in the modern world: dog poop. So, for the moment, let’s take my terms as a given. I’ve found a fair bit of heterogeneity in usage across academia and industry. The nomenclature above probably doesn’t line up with the terms that you heard in school or use in your ML app. One last point to call out on feature extractors is that they have a similar issue to the model server: they can be entangled with the process of actually gathering the raw data from the outside world. For a deeper dive into the difficulties of getting this right, check out my post on machine learning failures. Implementing feature extractors can be tricky even though it looks simple. Ideally, those two usages will just be two calls to the same feature extractor implementations, but nothing requires that. The astute reader will notice that we need feature extractors in both of the above subsystems: once when we learn the model and once when we evaluate the model. This is the portion of the application that takes raw data from some source and turns it into derived semantically meaningful data known as features. showing you which books you might want to read on Amazon). In many real world systems, this signal is often entangled with the portion of the application that actually takes the action (e.g. The output of this sub-application is at least some sort of signal about the action to take.
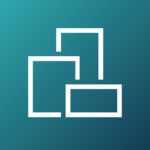
As inputs, it consumes features (more on them in a second) and the previously learned model. This is one of the less discussed parts of a machine learning application: whatever actually uses the model to make some sort of decision. It is typically, but not necessarily, a batch job that processes a data set consisting of training and possibly validation and/or test sets of instances. The model learning pipeline is typically what people think of when they think of an ML app. Machine Learning Applicationsįirst, when I say machine learning application (ML app), what do I mean? Actually, I mean several things. But in the spirit of starting before you’re ready, here’s my attempt to answer my own buring question.
#Lisence key chronos soho notes update#
What follows is a pretty rough initial analysis that I’ll probably update over time as engage more with the question.
#Lisence key chronos soho notes crack#
How do the principles of reactive systems relate to machine learning applications?įinding no answer on Google, I decided to give it a crack at answering it myself. So my obvious question after digging into this topic was: I use modern big data tools to build massive machine learning pipelines. Though I work at a web startup, I don’t personally build webapps.
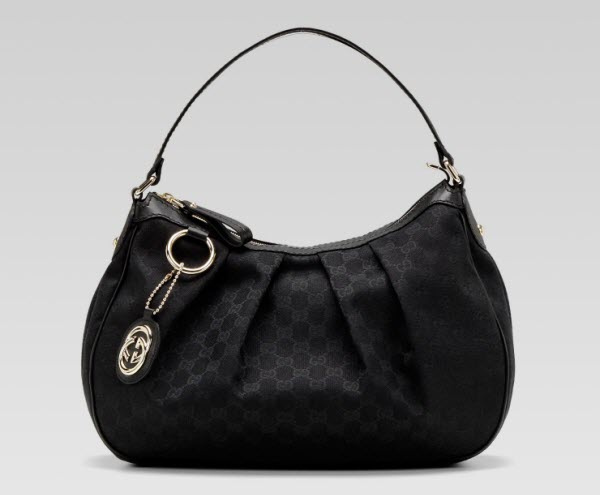
This is all exciting and engaging material, but I see one big oversight: machine learning. There’s even a very cool course on reactive programming you can take on Coursera.
#Lisence key chronos soho notes how to#
These ideas are building into a fairly substantial movement, and you can find books on how to apply the principles of reactive systems to issues in application development, domain modeling, web application development, and design patterns. It’s a succinct and revelatory distillation of many of the key strategies for fulfilling modern application requirements using sophisticated distributed systems design principles. For a brief introduction to the topic, I’d point you to the Reactive Manifesto.
#Lisence key chronos soho notes software#
Reactive systems are one of the most exciting developments in software development within the past several years.
